Wildlife conservation actions tend to be focused in specific locations because the data that inform them also tend to be highly localized.
But what if we could visualize where a species, or several, will be at any given time of the year, across vast — even continental — scales?
An artificial intelligence model developed by researchers at Cornell University hopes to do just that, showing it can deliver estimates of when and where birds occur across North America.
It can also give information on how different species of birds interact with each other and the environment.
“We can look not only at individual species but across entire communities, and pull in all sorts of information about what might be driving those species to occur there,” Courtney Davis, a research associate at the Cornell Lab of Ornithology, told Mongabay in a video interview.
“This has the potential to provide really high-resolution information to folks on the ground who are leading conservation, management and decision-making about biodiversity.”
In a study she co-authored and published in the journal Ecology, Davis details how researchers used billions of data points on bird sightings gathered by citizen scientists and birders, along with ecological data, to train the model.
With the help of “large observational and environmental data sets,” the team of researchers demonstrated how they estimated “landscape-scale species diversity and composition at continental extents.”
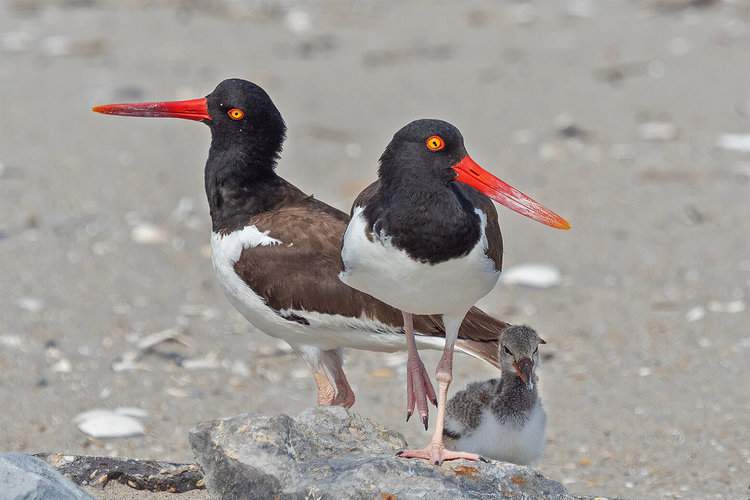
“One of our biggest challenges [was] to have robust and accurate measures of where and when species occur, and how many of them occur there,” Davis said. “It’s really technology that allows us to do this now and do it in a way that’s computationally efficient and at high spatial and temporal resolution.”
To get started, Davis and her team gathered bird observation data from eBird, an online database where birders can record their sightings. They used data on 500 bird species from North America provided by 900,000 birders. The model was then trained on various factors that included the presence and absence of species at a given location and time, along with environmental factors such as land cover, land-use patterns, proxies for urbanization like nighttime lights, elevation, and other measures of topography. To account for errors, the team also gathered information on who was gathering the data, and the duration and location of their birding activities.
“There is a measure that was developed here at the lab that allows us to account for differences in how people bird and their skill levels,” Davis said. “For example, if I go out and bird, and I’m not a very good birder myself, that’s very different from one of my colleagues going out who can identify everything in the area by sight or sound. This index allows us to correct or account for the differences in the behavior of birders.”
Any observational environmental data can be fed into the model as input. It then makes estimates about what species are likely to occur where. It also makes estimates about the likelihood of two or more species occurring together at the same time under the same environmental conditions, allowing researchers to create maps that identify areas that need to be prioritized for conservation purposes.
“With this approach, we get an additional layer of information that tells us something about how species are potentially interacting with each other across space,” Davis said. “We can also use it to think about how changes in the environment are likely to drive changes in that community structure and look at the impacts of those changes on that community.”
For instance, using the model, researchers at Cornell were able to observe and make predictions on when and where American wood warblers, a group of migratory birds, occur. The predictions aided their understanding of the community structure and how different bird species in the group interacted with one another.
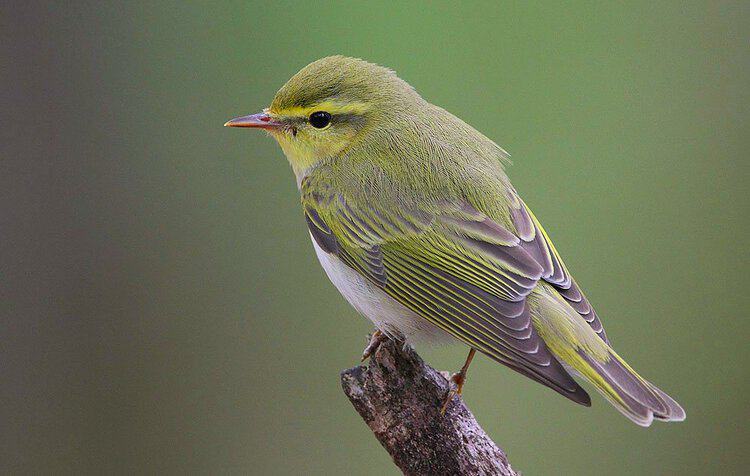
“We could see that their interactions in the breeding season are very different from their interactions in the migratory season or even nonbreeding season in that those species are actually more likely to co-occur strongly in the migratory period than in the breeding season,” Davis said. “You can actually start to disentangle how those interactions affect community structure throughout the full year, which is also pretty novel for this approach.”
At the moment, however, the model isn’t trained to estimate the number of birds at a given location. That, Davis said, is what her team is working on currently. However, it’s not an easy task, especially when it comes to training the model to make abundance estimates while also retaining its ability to characterize how different species interact with one another.
“One of the biggest challenges is thinking about how we model those interactions when we’re looking at counts, because in the ecological sense, when we say species interactions, it’s not so much interactions between individuals of a given species but more how these species are interacting in space and how their distributions overlap,” she said. “Characterizing that process is much more difficult when you’re thinking about abundance rather than estimating presence or absence.”
Even as her team works through those challenges, Davis said she’s hopeful the model can help conservation managers and researchers work more precisely to protect habitats and understand how factors like climate change might affect the distribution of bird species.
“We can look at the full annual cycle, but also at the implications of how those communities are structured across space,” she said. “Being able to generate that type of insight at continental extents, or even larger, is really exciting and unlocks a whole new level of potential analyses.”
Citation:
Davis, C. L., Bai, Y., Chen, D., Robinson, O., Ruiz‐Gutierrez, V., Gomes, C. P., & Fink, D. (2023). Deep learning with citizen science data enables estimation of species diversity and composition at continental extents. Ecology. doi:10.1002/ecy.4175
What you can do
Support ‘Fighting for Wildlife’ by donating as little as $1 – It only takes a minute. Thank you.

Fighting for Wildlife supports approved wildlife conservation organizations, which spend at least 80 percent of the money they raise on actual fieldwork, rather than administration and fundraising. When making a donation you can designate for which type of initiative it should be used – wildlife, oceans, forests or climate.
This article by Abhishyant Kidangoor was first published by Mongabay.com on 8 November 2023. Lead Image: A male Allen’s hummingbird (Selasphorus sasin). Image by Andrej Chudý via Flickr (CC BY-NC-SA 2.0).
Leave a Reply